Asif Khan
Postdoctoral Fellow Harvard Medical School.
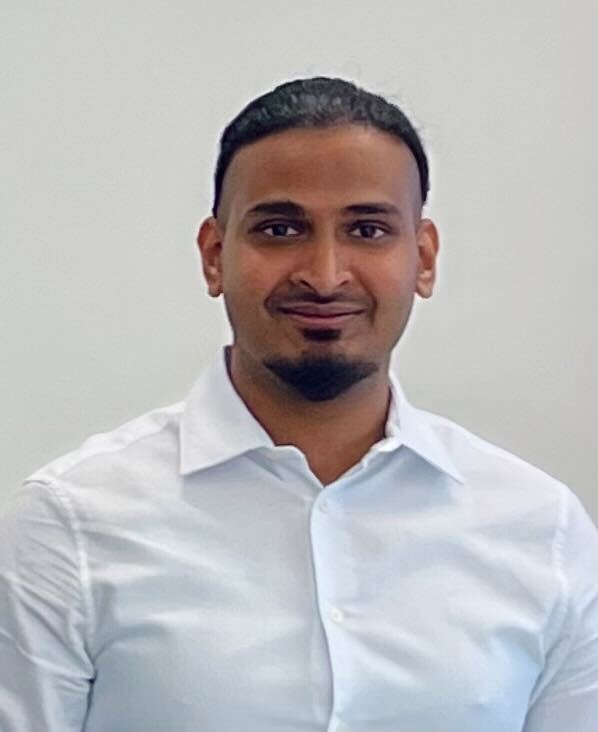
I am a postdoctoral fellow at Harvard Medical School in Prof. Chris Sander’s Lab. My research focuses on machine learning methodologies for cancer risk prediction and combination therapy, with a particular emphasis on interpretable approaches that can guide clinical decision-making.
For cancer risk prediction, I develop deep learning models using large-scale longitudinal electronic health records. This involves two main components:
-
Representation Learning: Encoding patient histories into continuous feature representations with key properties such as disentangled risk factors, interpretability of encoded representation, and mitigating population-specific biases.
-
Risk Prediction: Discrete-time event models for cancer risk, with particular emphasis on modelling uncertainties to ensure robust performance and generalisation across diverse patient populations, including out-of-distribution scenarios.
In combination therapy, the goal is to develop computational models of cell biology that accurately capture cellular behaviours and underlying biological processes. To this end, I use machine learning to learn differential equation models that can predict molecular responses to new drug combinations. These models are used for designing effective combinatorial therapies and optimising experimental design.
I have a PhD from the University of Edinburgh, advised by Prof. Amos Storkey in the Bayesian and Neural Systems research group. My doctoral research was at the intersection of geometry and deep representation learning. Please look at my publications for further insights into my work and research.
Selected Publications
- Adversarial robustness of VAEs through the lens of local geometryInternational Conference on Artificial Intelligence and Statistics (AISTATS) 2023
- Toward real-world automated antibody design with combinatorial Bayesian optimizationCell Reports Methods 2023
- HALO: HAmiltonian Latent Operators for content and motion disentanglement in image sequencesAdvances in Neural Information Processing Systems (NeurIPS) 2022